Your business outcomes drive our innovation
Proven custom software product engineering solutions for industry leaders
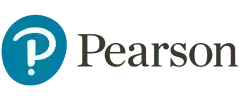

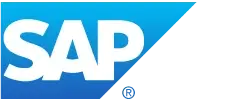
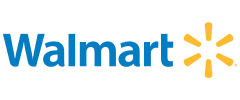
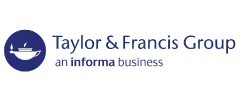
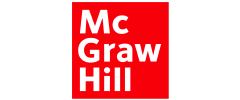
Proven custom software product engineering solutions for industry leaders

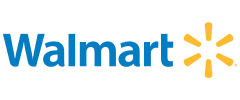
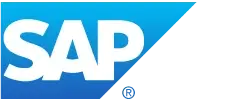
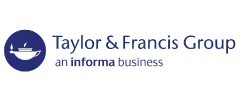
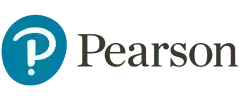
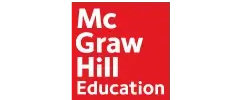
Your business outcomes drive our innovation
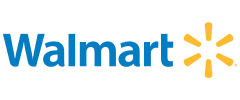

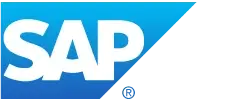
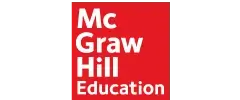
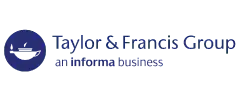
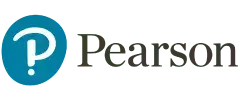
Next-Generation AI solutions
Tricon designs and implements cutting-edge AI, Machine Learning, and Large Language Model solutions that are private, secure, and tailored to individual situations.
Rather than relying on only a single, isolated solution, Tricon combines the best Generative AI and observational logic tools into applications that scale across your organization’s workflows.
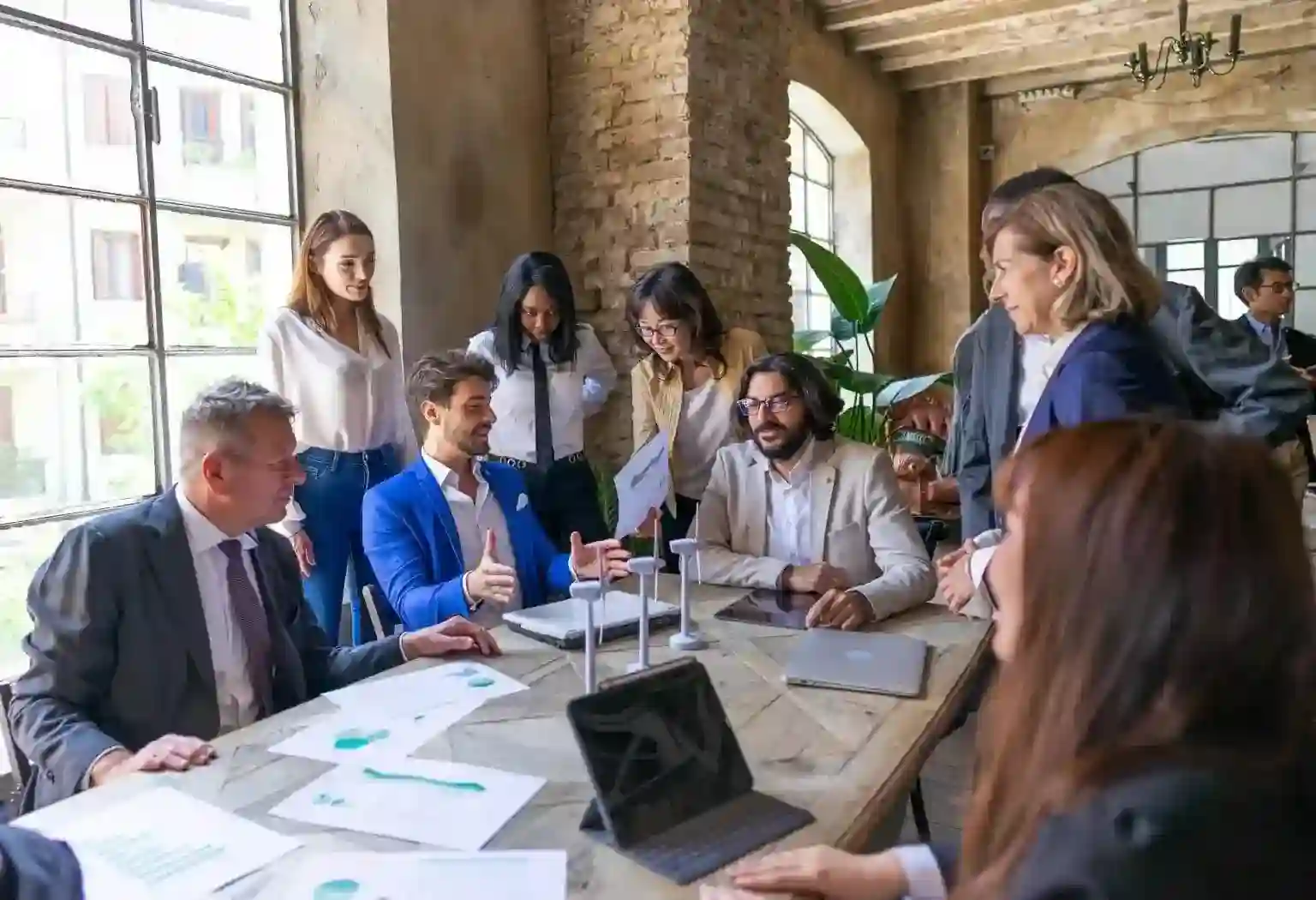
Custom software for successful organizations
We work collaboratively with our clients to create products that deliver immediate value and long-term progress to achieve their goals.
Learn why industry leaders like SAP, Merck, Walmart, McGraw-Hill Education, Pearson, and Taylor & Francis partner with Tricon Infotech for sustained innovation.
Building Generative Relationships
Research firm Gartner® has published a new case study, “Building Generative Relationships (McGraw Hill and Tricon),” that explores the partnership between educational publisher McGraw Hill and custom software development company Tricon Infotech.
Gartner characterizes generative relationships as those in which:
“Successful organizations have created new business and significant value by forging relationships that generate high-impact business outcomes because they work with each other to achieve these results, rather than hiring tech firms to work for them.”
Learn more about the case study in this press release.
The Tricon Infotech difference

Deep Experience
We have over a quarter-century of experience building custom software products for a wide range of industries. That means we can hit the ground running.

Speed to value
Our unique teams model and engagement model builds and deploys software products faster and with less code, using iterative solutions for rapid progress.

Business continuity
We carefully study and understand your technical environment and deploy them without disrupting your ongoing business. The result is innovation without undue risk.

Business intelligence
We infuse data management and analytics into all our products, giving you a comprehensive, birds-eye view from day one.

Structured for agility
Our ideal size allows us to operate nimbly and efficiently and offer you unparalleled access – up to and including at the C-level.

Dedicated teams
We build dedicated, high-performing teams of expert engineers for each client. Gain experienced staff without the delay, hassle, or risk.
Building Generative Relationships
Tricon Infotech builds generative relationships by investing alongside our clients to deliver mutual value and growth.
A landmark case study from Gartner studying McGraw Hill Education’s work with Tricon observes:
The two parties have created a generative relationship based on deep skills and mutual commitment. Tricon has invested and continues to invest in deep business and technical skills which gives them a competitive differentiation from more staff augmentation vendors. McGraw Hill gains value from that investment through working with Tricon in some of its most important initiatives and strategies.
– Gartner, ”Building generative relationships: McGraw Hill and Tricon” (2023)
Our partner engagements
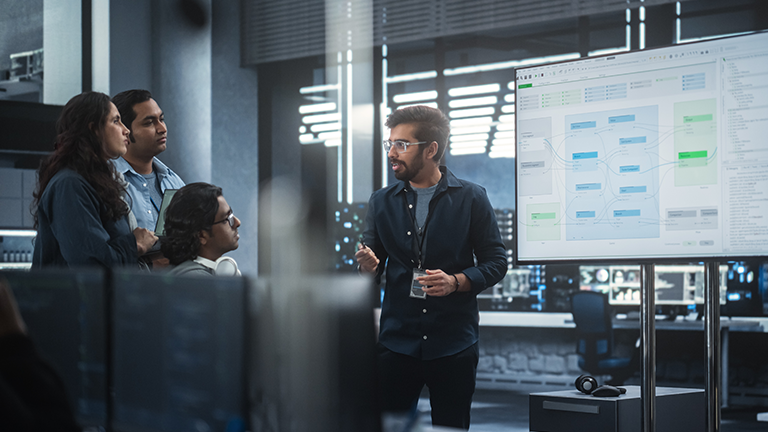
-
- Posted by Adi Chikara
Understanding how to deploy Generative AI is the key to unlocking its transformative power.
Read More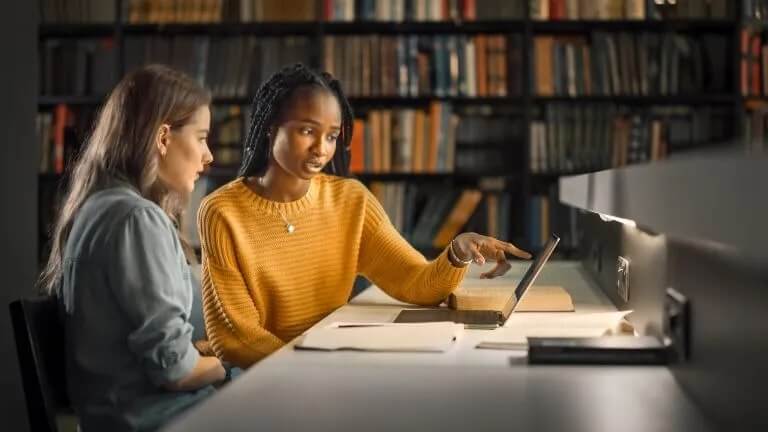
-
- Posted by Tricon
A book publisher integrated data analytics into its ebook platform to increase insights and sales.
Read More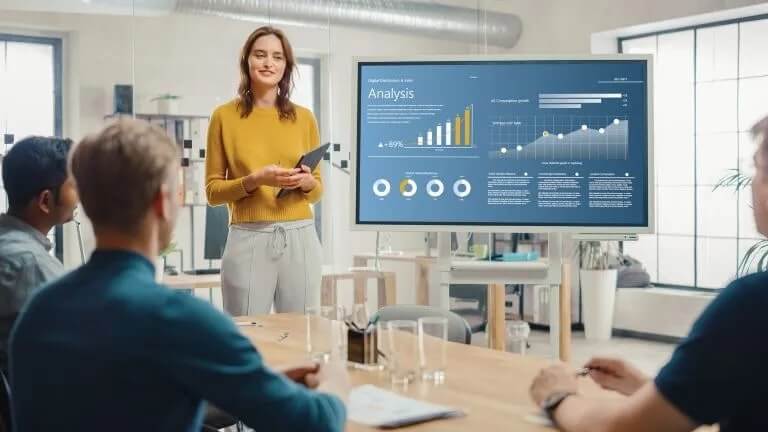
-
- Posted by Tricon
A robust data warehouse turned an enormous flow of information into valuable insights.
Read MoreYour goals drive our innovation

Custom software products
Our cross-functional teams build products that put a smile on people’s faces, drive revenue, improve efficiency, and increase company value.

AI/Machine Learning tools
Our engineers harness the power of AI, machine learning, and Large Language Models to enhance user experiences in a reliable, structured, and secure manner.

Accessibility
We build accessible products from inception, rather than doing costly retrofits later. That means better user experiences and staying on the right side of the law.

Analytics & Business Intelligence
Tricon bakes data collection and analytics into its products, along with the infrastructure to store and analyze it, meaning actionable insights from day one.

Complete digital transformations
Say goodbye to disconnected systems, outmoded manual processes, clunky reports, and poor experiences. Tricon turns disparate components into seamless systems.
Industries we serve

Publishing
- Full digital transformations
- Catalogues and data management
- Back-end inventory and fulfillment
- Sales and marketing solutions
- eBook and online journal hosting

EdTech
- Comprehensive curriculum solutions
- Custom Learning Management Systems
- Interactive learning experiences
- Online custom publishing

Finance
- Analytics and Business Intelligence
- Automated data processing
- Rules-based risk assessments
- Compliance tools
Industries we serve

Publishing
- Full digital transformations
- Catalogues and data management
- Back-end inventory and fulfillment
- Sales and marketing solutions
- eBook and online journal hosting

EdTech
- Comprehensive curriculum solutions
- Custom Learning Management Systems
- Interactive learning experiences
- Online custom publishing

Finance
- Analytics and Business Intelligence
- Automated data processing
- Rules-based risk assessments
- Compliance tools
Life at Tricon
Tricon Infotech offers unparalleled opportunities to learn and grow thanks to direct access to senior leaders, willing and enthusiastic mentors, and exposure to a wide range of technologies while building products of the future. See how current employees have furthered their knowledge, skills, careers, and lives at Tricon.
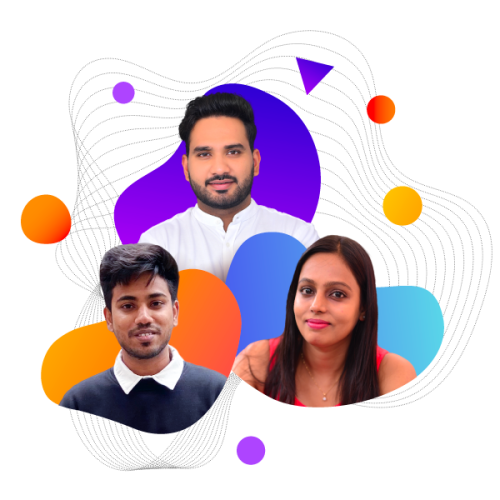